PhenoDigm
PhenoDigm is an algorithm to prioritise disease gene candidates based on phenotype information. It incorporates the OWLSim mechanism to align ontological descriptions and generate a similarity measure.
PhenoDigm is an algorithm to prioritise disease gene candidates based on phenotype information. It incorporates the OWLSim mechanism to align ontological descriptions and generate a similarity measure.
Analyzing curated phenotype annotations to associate animal models with human diseases
Model organisms represent a valuable resource for the characterisation as well as identification of disease-gene associations, especially where the molecular basis is unknown and there is no clue to the candidate gene’s function, pathway involvement or expression pattern. To systematically apply this methodology, PhenoDigm uses a semantic approach to map between clinical features observed in humans and mouse and zebrafish phenotype annotations. The database allows browsing/searching of genetic diseases from the Online Mendelian Inheritance in Man (OMIM), DECIPHER and Orphanet databases and display of the resulting animal model matches ranked by their phenotypic similarity to the disorder. To date, phenotyped mutants from the Mouse Genome Informatics Database (MGI), the Sanger Mouse Genetics Project (MGP) and the Zebrafish Model Organism Database (ZFIN) are incorporated. Future builds will incorporate data from projects performing high throughput phenotyping of every protein-coding gene: the International Mouse Phenotyping Consortium (IMPC) and Zebrafish Mutation Project (ZMP).
Linking tissues to phenotypes using gene expression profiles
Despite great biological and computational efforts to determine the genetic causes underlying human heritable diseases, approximately half (3500) of these diseases are still without an identified genetic cause. Model organism studies allow the targeted modification of the genome and can help with the identification of genetic causes for human diseases. Targeted modifications have led to a vast amount of model organism data. However, these data are scattered across different databases, preventing an integrated view and missing out on contextual information. Once we are able to combine all the existing resources, will we be able to fully understand the causes underlying a disease and how species differ.
Here, we present an integrated data resource combining tissue expression with phenotypes in mouse lines and bringing us one step closer to consequence chains from a molecular level to a resulting phenotype. Mutations in genes often manifest in phenotypes in the same tissue that the gene is expressed in. However, in other cases, a systems level approach is required to understand how perturbations to gene-networks connecting multiple tissues lead to a phenotype. Automated evaluation of the predicted tissue-phenotype associations reveals that 72-76% of the phenotypes are associated with disruption of genes expressed in the affected tissue. However, 55-64% of the individual phenotype-tissue associations show spatially separated gene expression and phenotype manifestation. For example, we see a correlation between 'total body fat' abnormalities and genes expressed in the 'brain', which fits recent discoveries linking genes expressed in the hypothalamus to obesity. Finally, we demonstrate that the use of our predicted tissue-phenotype associations can improve the detection of a known disease-gene association when combined with a disease gene candidate prediction tool. For example, JAK2, the known gene associated with Familial Erythrocytosis 1, rises from the seventh best candidate to the top hit when the associated tissues are taken into consideration.
Using association rule mining to determine promising secondary phenotyping hypotheses
The International Mouse Phenotyping Consortium (IMPC) systematically phenotypes every protein-coding gene in the mouse. The phenotype screens are executed based on standard operating procedures (SOPs) in different contributing research institutes, such as the Wellcome Trust Sanger Institute or the MRC Harwell institute. In the initial phase of the IMPC project, a subset of phenotype screens -- the primary phenotype screens -- are assessed providing a limited phenotypic description of the mice that have been mutated. However, mice that show interesting phenotypes in the primary screens will be assessed in further secondary and tertiary SOPs.
To further help with the screening procedures, we applied a data mining approach to existing literature-curated phenotypes to predict secondary phenotype candidates from the phenotypes that have been assigned to mutants in the primary screen. We used an association rule mining approach that extract dependencies between phenotypes based on an annotation set. While association rule mining was traditionally applied to supermarket transactions to find items that have been frequently purchased together, it already found application in the biological domain [1,2]. We treated existing phenotype annotations for genes as a transactions and determined patterns of co-occurring phenotypes. These patterns were then used to predict potential secondary phenotypes based on the phenotypes assigned in the primary screens. We excluded relations that have been established already and provide the remaining as predicted secondary phenotypes here.
Archive Page
This page is maintained as a historical record and is no longer being updated.
This is an Archive page and is no longer being updated. It is being maintained as a historical record of the work of the Wellcome Sanger Institute.
Downloads
Disease-gene association data with their prioritised models can be accessed from https://monarchinitiative.org/.
Phenotype-tissue associations from gene expressionprofiles can be download as the raw data from the FTP site.
Sanger Institute Contributors
Previous contributors
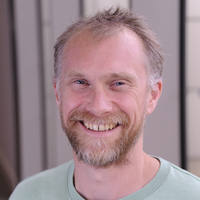
Dr Jules Jacobsen
Senior Software Developer
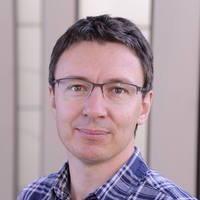
Dr Damian Smedley
Former Senior Scientific Manager