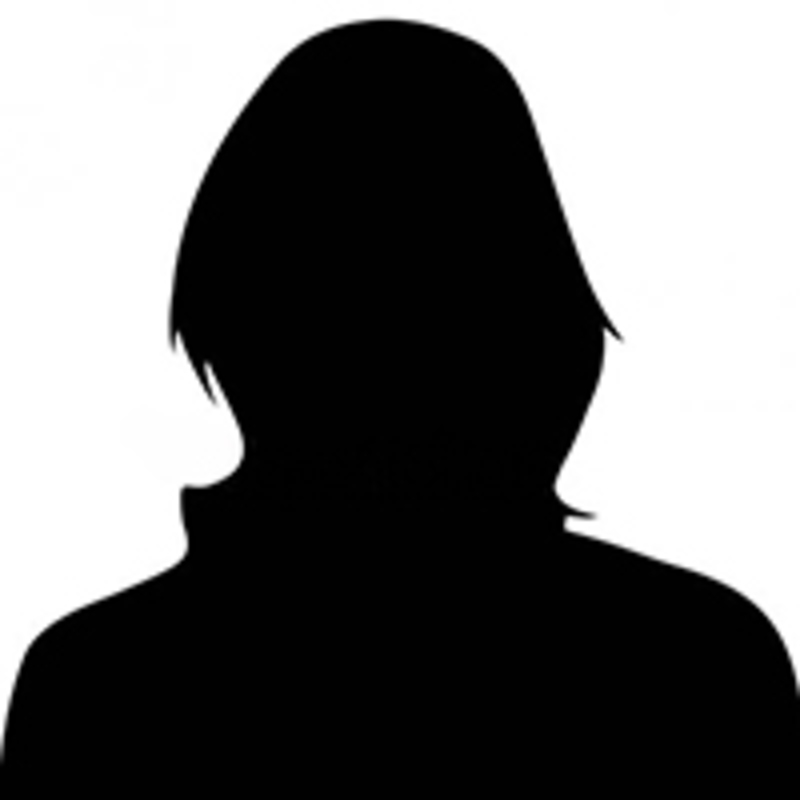
Bioinformatician, Cellular Genetics Informatics
I’m a Bioinformatician with a strong foundation in transcriptomics (bulk RNA-Seq and Visium)
Education:
I earned my bachelor’s degree in Applied Mathematics and Physics from the Moscow Institute of Physics and Technology. My studies gave me a strong foundation in mathematical modeling, computational techniques, and the physical principles that I now apply to solve biological problems.
Research Interests:
I’m passionate about developing tools and workflows for the analysis of biological data, making it easier for researchers to extract meaningful insights from complex datasets.
Skills and Tools:
I rely on a diverse toolkit to support my research:
- Nextflow: For robust and reproducible pipeline development.
- Python: For data analysis, machine learning, and visualization, including libraries like Pandas, PyTorch, and Matplotlib.
- Containerization (Docker, Singularity): To ensure reproducibility across environments.
- ML and DL: Experience in classical machine learning and natural language processing
Through my work, I aim to make biological data analysis more efficient and reliable, contributing to research that has a lasting impact.
Research Experience:
- Sanger Institute: I’m currently working at the Wellcome Sanger Institute, where I focus on developing pipelines for the analysis of Single Cell data
- Skoltech: While at Skoltech in the Philipp Khaitovich group, I studied the prefrontal cortex of humans, chimpanzees, and macaques using 10x Genomics Visium data. My work involved identifying genes with human-specific expression patterns
- EMBL-EBI: At EMBL-EBI, I developed and validated the CellTypeScanner tool, combining Nextflow and R to streamline cell type classification workflows.
- BostonGene: I worked on identifying subtypes of cervical cancer using RNA-seq data and linked them to patient survival and immunotherapy outcomes. I also developed a method to apply qPCR-like scoring to RNA-seq data and built machine-learning models to predict neuroendocrine prostate cancer.
My timeline
Bioinformatician, Cellular Genetics Informatics, Wellcome Sanger Institute
Graduated with Bachelor degree, Moscow Institute of Physics and Technology
Intern Researcher, Philipp Khaitovich group, Skoltech
Intern Bioinformatician, Gene Expression group, EMBL-EBI
Junior Bioinformatician, BostonGene
Intern Bioinformatician, BostonGene