Lehner Group
Programmable biology
Our Approach
Molecular biology and genomics have given us a good description of the parts of biological systems and some understanding of how they cause disease when they fail. However, we are still very limited in our capacity to predict how biological systems respond to change – even simple changes such as altering one letter in the DNA sequence of a gene associated with disease. And we lack the knowledge to easily engineer biology in the same way that we can engineer cars or computers.
We seek to build the foundations of knowledge needed to deliver programmable biology. Our approach is to use massively parallel DNA synthesis, selection and sequencing experiments to generate very large datasets of how changes in DNA sequence alter the properties of proteins, RNAs and cellular networks. We then use these data in combination with mechanistic modelling and machine learning to derive mechanistic insights and to build predictive and generative models.
We favour experimental methods that report closely on defined molecular phenotypes directly encoded by sequence. This approach allows us to make measurements at sufficient scale to infer the underlying biophysical consequences of genetic changes.
In particular, we focus on combining genetic changes within DNA and making multiple molecular measurements using the same variant libraries to generate data at scale. To achieve this we actively design and refine deep mutational scanning experiments (also known as multiplex assays of variant effect (MAVEs)). We also develop and hone a range of computational methods to analyse the data, to extract mechanistic insights and to build predictive models.
Protein folding and solubility
Many changes in DNA sequence cause disease because they reduce the stability of the encoded proteins. We seek to generate the data and predictive models needed to understand how and predict which changes alter protein stability. Our ultimate aim is to provide the toolkit of knowledge needed to bioengineer these changes directly.
Our team is developing, benchmarking and applying at scale experimental methods to quantify the effects of hundreds of thousands of mutations on the abundance and solubility of thousands of proteins. Our work will explore a wide range of proteins across the human proteome and those found in other organisms across the Tree of Life.
Our aim is to generate reference datasets for the clinical interpretation of genetic changes. In addition, our work is designed to generate improved understanding of, and models to predict, the effects that DNA changes produce. This will generate new opportunities to engineer proteins as therapeutics and deliver biological engineering.
Molecular interactions and networks
Nearly all proteins and RNAs function by specifically binding to other molecules. To truly understand molecular biology and enable effective drug development, it is vitally important that we are able to understand, predict and engineer binding affinity, specificity and kinetics of proteins in situ.
We believe that by generating data at scale we can directly tackle these central problems of molecular biology. We are developing and using methods to quantify the binding of huge libraries of protein variants to other proteins and macromolecules, as well as their binding to small molecules and drugs.
In addition, the structures of many proteins are constantly changing within the cell, adding further complexity. While some regions of proteins adopt well-folded globular structures, others are more dynamic, adopting ensembles of structures. Our work will help us to understand molecular recognition across protein diversity, including how interaction affinity and specificity are encoded in intrinsically disordered, dynamic protein regions.
Allostery and regulatory control
The transmission of information from one site in a protein to another – allostery – is central to nearly all biological regulation. It is so important that Jacques Monod referred to it as the ‘second secret of life’. Indeed, many of the most effective therapeutics work by binding to allosteric sites.
However allostery is still poorly understood and difficult to predict, and most proteins do not have any known allosteric sites. We believe that defining the position and action of all the allosteric sites present on every protein would revolutionise drug development.
To help achieve this goal we have developed a general approach to identify allosteric sites that can be applied to many different proteins. The approach combines mutational scanning with machine learning and has already demonstrated its effectiveness by allowing us to build the first comprehensive maps of allosteric communication. Applying these approaches to study the receptors and proteins associated with cancer will help us understand how they work and identify sites that could be targeted to inhibit or control their activity.
In addition, we expect that generating data at scale will allow the global research community to develop new methods to accurately predict and engineer allosteric control sites in proteins.
Amyloids and protein aggregation
Amyloid fibrils are remarkable alternative structures of proteins and are the pathological hallmarks of more than 50 human diseases, including Alzheimer’s disease, Parkinson’s disease, motor neurone disease(amyotrophic lateral sclerosis) and systemic amyloidosis. Changes in the proteins that aggregate as amyloids in the common forms of neurodegeneration also cause rare familial neurodegenerative diseases.
In collaboration with the lab of Benedetta Bolognesi we have developed high-throughput methods to quantify the aggregation rates of tens of thousands of proteins at the same time. We are now applying these methods at scale to identify all of the sequence changes that cause human proteins to aggregate to cause disease.
It is very difficult using current methods to study the transient states that initiate protein aggregation and the formation of amyloids. Yet understanding these structures and processes is fundamental to developing molecules that could treat and prevent these devastating diseasese. So we are developing more complicated combinatorial mutagenesis experiments to explore and map the processes and transient states that initiate protein aggregation and amyloid formation.
RNA processing and gene expression
In addition to directly controlling protein structure and action, changes in DNA can also cause disease by affecting how proteins are produced.
DNA is first transcribed into RNA and these RNA strands are extensively processed before they are translated into proteins. Defects in RNA processing are a common cause of genetic disease, particularly the splicing out of intervening intronic (non-coding) RNA sequences. In collaboration with the lab of Juan Valcárcel, we are using massively parallel assays to quantify, understand and predict how changes in sequence alter how human mRNAs are spliced.
A second common cause of genetic disease is the introduction of stop codons into mRNAs. In collaboration with the lab of Fran Supek, we are generating data at scale to better understand how, and predict which, DNA changes produce this effect.
In addition, all not stop codons are equally effective. Some result in the destruction of RNA through a process called nonsense-mediated mRNA decay (NMD), while others do not. We want to understand how, and predict which, stop codons trigger NMD. We hope that this knowledge will allow researchers to design drugs that could treat a wide range of diseases by tricking cells to ignore stop codons.
Evolution and engineering
The combinatorial complexity of biology puts fundamental limits on how we can understand and engineer it. For example, there are more ways to make a small protein of just 100 amino acids (20^100 =~10^130) than there are atoms in the universe. As a result, we will never be able to experimentally (or computational) study even a tiny fraction of these possibilities.
Therefore, if we wish to engineer biology and to understand evolution, we need to develop accurate generative models to predict activity from sequence. To achieve this we are using diverse experimental approaches to ask the following questions about the genetic architecture of proteins and RNAs:
- How many different sequences can encode a particular function?
- To what extent and why do the effects of DNA changes alter as a protein evolves?
- Can we build simple and sparse models to predict what happens when one, a few or many DNA changes are combined?
Our expectation is that the answers to the questions will help us to understand how proteins and mRNA evolve, and to generate data at scale to train and test generative models for bioengineering.
Core team
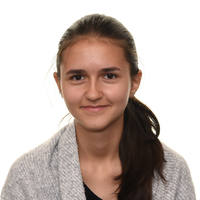
Dr Anna Arutyunyan
Postdoctoral Fellow
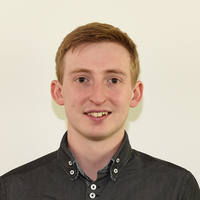
Mr Alexander Dove
Advanced Research Assistant
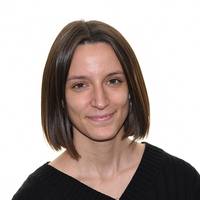
Carla Folgado Salido
PhD Student
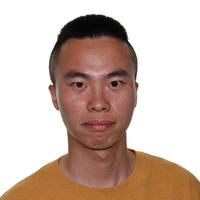
Dr Xinhao Hou
Postdoctoral Fellow
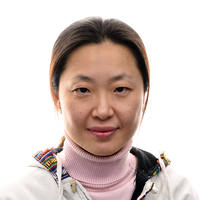
Dr Xianghua Li
Senior Staff Scientist
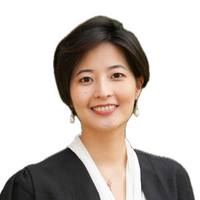
Xiaotian Liao
PhD Student
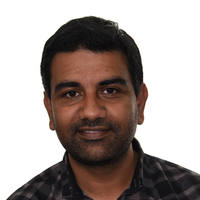
Dr Mohsin M. Naqvi
Postdoctoral Fellow
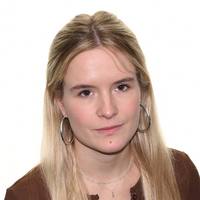
Aina Marti Aranda
PhD Student
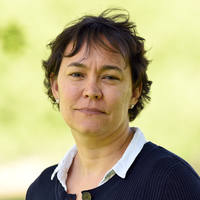
Dominique Von Schiller
Advanced Research Assistant
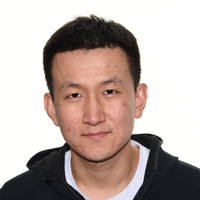
Mr Huijin Wei
Advanced Research Assistant
Related groups
Partners
We work with the following groups
External
Dr Benedetta Bolognesi
Protein Phase Transitions in Health and Disease Group, IBEC (Institute for Bioengineering of Catalonia)
External
Professor Fran Supek
Genome Data Science group, IRB (Institute for Research in Biomedicine, Barcelona)
External
Professor Juan Valcárcel
Regulation of Alternative pre-mRNA Splicing during Cell Differentiation group, CRG (Centre for Genomic Regulation, Barcelona)